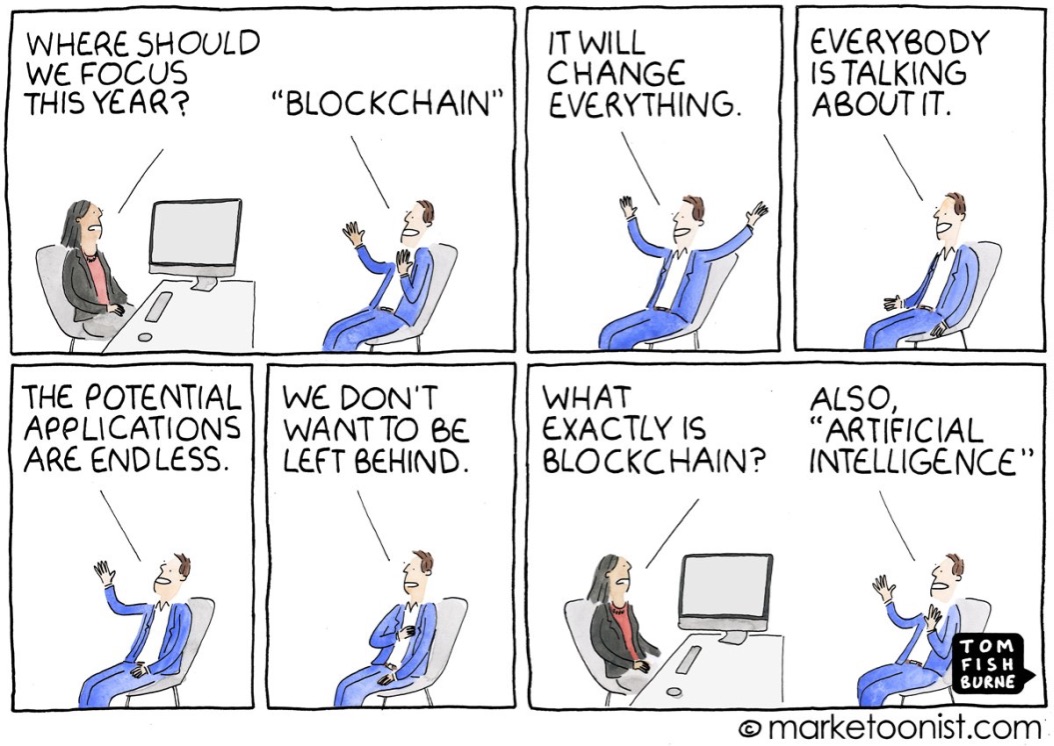
Big data. Blockchain. And now, Artificial Intelligence. Mention these two words to a group of hard-core data scientists and you will more than likely elicit giggles. Yes, AI has become the biggest buzzword these days.
But don’t let that fool you – AI is real, and it is revolutionary. It is already disrupting many industries and we have only scratched the surface. As a business leader, you know you can’t ignore it. But how do you figure it out with so much hype and vaporware out there?
Before you run off and enrol in a machine learning class, here’s the good news: you don’t need to be an expert to harness the power of AI. But you do need to understand enough to have a vision for what AI can do for your business today – and the possibilities it presents for the future.
What AI are we talking about?
Simply put, artificial intelligence is when a machine mimics the cognitive functions of the human brain, such as learning and problem solving. This is far from a new concept; it’s been 75 years since Turing proposed that a digital computer can simulate any process of formal reasoning. So why all the sudden buzz about AI?
The first reason is data. Historically, data was the biggest constraint to building useful artificial intelligence; data was expensive to store, and slow to process. In the last few years, we’ve reached the tipping point where data has ceased to be the constraint – so much, in fact, that today the constraint has shifted to the imagination. If you can imagine it, chances are it’s not that far from possible.
The second is proof points. We have all experienced useful versions of AI that have surprised and delighted us. Think of that feeling the first time you used Alexa or Siri. Or the first time you used Waze to get home in rush hour. Different forms of AI have become ubiquitous in our lives, and that has powered the demand for more.
There are many branches of artificial intelligence, and the scope of AI itself is a hotly debated topic. For the purpose of this article, I’m going to focus on two that will be powerful for your business: machine learning and natural language processing.
Machine learning refers to computer algorithms that improve automatically through experience. In other words, as new information presents itself, the computer learns and adapts. Deep learning is a subset of machine learning based on artificial neural networks that act very much like the human brain. Think about the complexity that lies behind a completely autonomous vehicle, or a computer program that can beat the world champion at Go. That is deep learning.
Natural language processing (NLP) is what gives machines the ability to read and understand the human language. This involves speech recognition, natural language understanding, and natural language generation. Virtual assistants and chat bots are probably the most prominent examples of NLP today.
Leveraging AI to transform your business
Okay, enough of the tutorial; how can AI help your business? Before I left the corporate world to start Payson Solutions, I built a world-class data science team for a leading financial services firm. I was, and still am, far from an expert in AI, but I have a passion for leveraging advanced analytics and the vision to bring solutions to life. From my own experience, here are the four things to consider as you figure out how to leverage AI to transform your business:
- It’s all about the use case. Don’t treat AI like a hammer looking for a nail; build AI solutions for your biggest business challenges.
- Point your AI investment to the present… and the future. Think of AI and its possibilities across all three horizons of growth
- Build, buy, or both? Great AI is created by exceptional human talent, and there is not enough to go around these days.
- Execution is just as important as the science. Getting product to market is not always easy; don’t let your great AI solutions die on the vine.
It’s all about the use case
Unless you’re Google, chances are AI isn’t your business. So, don’t chase after AI for the sake of AI. What is your core strategy? What are your business goals? What are your biggest challenges? Work back from those and then figure out what role, if any, AI can play.
In the financial services industry, I tended to think of use cases in 3 buckets:
- More powerful predictive models that generate hard dollars in value. A perfect example of this is a Fraud model for credit card purchases, built on a neural network using machine learning.
- Real-time, personalized experiences that create loyalty. Think of a robo-advisor who can provide investment recommendations completely customized to your needs.
- Automation of manual processes that saves operating costs. A good example of this is quality control; can you leverage NLP to replace humans doing expensive (and error-prone) reviews of customer calls for compliance purposes?
Whatever your business model is, the first thing you should always ask when someone suggests an AI project is, “what’s the business case?” If you can’t articulate one, move on – unless you’re the business leader I’ve never met who has a lot of spare time on their hands.
Point your AI investment to the present… and the future
When it comes to AI, or the broader topic of innovation in general, people often ask me, “Should we focus on ideas we can implement today, or think bigger?” My answer? “Both!”
AI is here; I’d be very surprised if there isn’t a solution for your business that is both feasible today and beneficial to your business, in terms of either your CX or your bottom-line. But like Wayne Gretzky says, you also need to skate to where the puck is going. If you’re not looking out past today to your second and third planning horizons, you run a big risk of falling behind in the future.
At my last company, I built an innovation lab in the technology hub of Kitchener-Waterloo for just this purpose. By putting the lab in a different city, away from the meeting culture at head office, they were able to focus on swinging for home runs, while the business continued to knock out singles. We went in with our eyes wide open that not all of the projects they worked on would make it to market, but the ones that did were game-changers.
Build, buy, or both?
A lot of larger companies (you know who you are!) are throwing a lot of money at AI start-ups, hoping to find something that sticks. With AI being such a hot topic, there appears to be an irrational fear of missing out (FOMO) going on. But like I said, AI is here to stay, so you should really be thoughtful about how to plan for it and start building your internal capabilities today.
There are two massive challenges companies face: data and talent. To build AI, you need massive amounts of data that is clean, accessible and ready to be processed. Many companies that haven’t had a data culture have not designed their systems to store historical data that is essential for AI. And if they have stored it, it is often in disparate systems or databases that don’t talk to each other. Doing the upfront heavy lifting to get your data layer in shape is foundational to being able to build AI solutions.
Just as important as data but often overlooked is talent. There are a lot of people calling themselves data scientists today, but the true rock stars – those who can code, model, and move at the pace of business – are practically unicorns. And even if you can find them, good luck keeping them. I had great success recruiting data scientists from the engineering program at the University of Waterloo, but I found it increasingly hard to retain them, especially with the draw towards Silicon Valley and the weak Canadian dollar.
There are lots of companies out there professing they can help you bridge the gap between buy and build, but do your diligence as I have found many of them to be pretenders. One that I trust is a former colleague of mine, Priya Dwivedi, who founded Deep Learning Analytical Solutions and has helped many companies who don’t have internal teams with their AI solutions.
Execution is just as important as the science
You have an awesome new chatbot you’ve built off the side of your desk that will revolutionize your CX. You need the engineers to help you get it into production, but they’re too busy dealing with back-end problems and platform modernization. Sound familiar?
In my 20 years working in large companies, I saw more great ideas struggle to get to market than I care to count. Different departments have different priorities. Unexpected fires need to be put out. Everybody’s already trying to do too much. How can you think about AI in these conditions?
These aren’t easy problems to solve, but I would offer these tips:
- Focus – pick one AI project at a time and go all-in on it.
- Have a strong executive sponsor – pet projects started by the analytics team rarely succeed without active business sponsorship.
- Put a cross-functional team on it – having both intent and execution co-located and working hand in hand on the project will help it be implemented with quality.
A final word of advice: like any other tool, with AI, work back from “for the sake of what?” Because as powerful as AI is, it is just a tool, and it’s only effective if it’s solving your biggest challenges.
Share your thoughts